“PoPGAN: Points to Plant Translation with Generative Adversarial Network” by Yamashita, Akita and Morimoto
Conference:
Type:
Entry Number: 48
Title:
- PoPGAN: Points to Plant Translation with Generative Adversarial Network
Presenter(s)/Author(s):
Abstract:
We propose a method of 2D image generation for plant images and animations, based on deep learning. Our point-input interface allows users to specify the positions of leaves in a simple manner. Our neural network enables the generation of high-quality images and animations, using feature interpolation.
Existing image generation methods based on deep learning have problems with the input of image descriptions. Methods with input labels or parameters force users to prepare quite sensitive drawings or excessively rough specifications. Moreover, to generate animations, far more inputs are required.
The generative adversarial networks (GANs) [Goodfellow et al. 2014] method is one of the most effective methods for image generation. The conditional GANs (cGANs) [Mirza et al. 2014] method allows contents to be specified by inputting numerical values or labels. AriGAN [Giuffrida et al. 2017], which specializes cGANs for generating plant images, can specify the number of leaves only. With pix2pix [Isola et al. 2017], which applies cGANs with a color-coded label image as input, it is sometimes necessary to specify the target shape in detail.
In this work, we propose a two-stage deep learning model which generates high-resolution plant images and animations using relatively little training data, and point-input method, to facilitate simple input.
Keyword(s):
Additional Images:
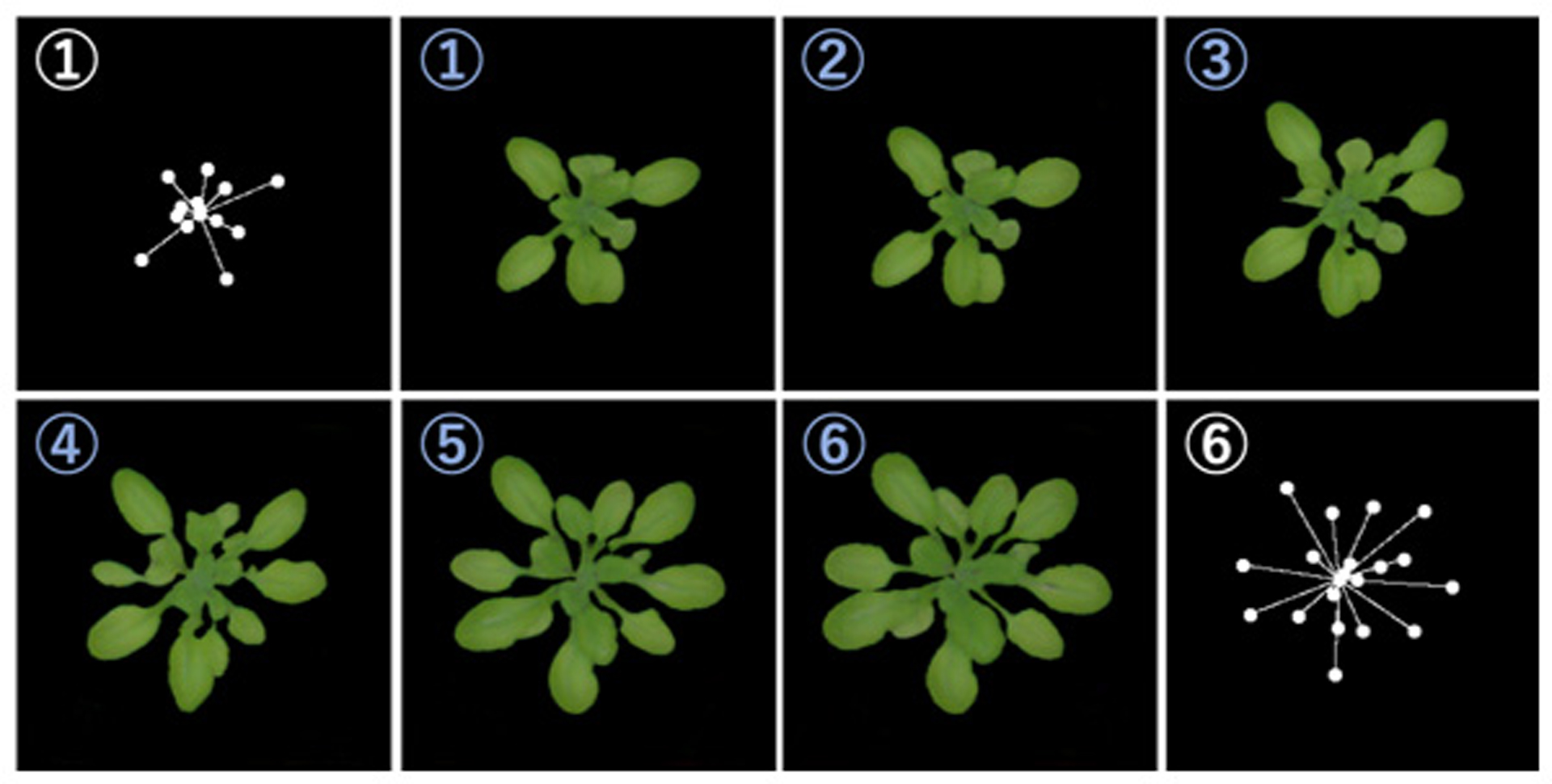