“OceanGAN: A Deep Learning Alternative to Physics-Based Ocean Rendering” by Ratto, Szeto, Slocum and Del Bene
Conference:
Type(s):
Entry Number: 89
Title:
- OceanGAN: A Deep Learning Alternative to Physics-Based Ocean Rendering
Presenter(s)/Author(s):
Abstract:
Physics-based models for ocean dynamics and optical raytracing are used extensively for rendering maritime scenes in computer graphics [Darles et al. 2011]. Raytracing models can provide high fidelity representations of an ocean image with full control of the underlying environmental conditions, sensor specifications, and viewing geometry. However, the computational expense of rendering ocean scenes can be high. This work demonstrates an alternative approach to ocean raytracing via machine learning, specifically Generative Adversarial Networks (GANs) [Goodfellow et al. 2014]. In this paper, we demonstrate that a GAN trained on several thousand small scenes produced by a raytracing model can be used to generate megapixel scenes roughly an order of magnitude faster with a consistent wave spectrum and minimal processing artifacts.
References:
- Berk, P. Conforti, R. Kennett, T. Perkins, F. Hawes, and J. van den Bosch. 2014. MODTRAN6: a major upgrade of the MODTRAN radiative transfer code. In Proc. SPIE 9088, Algorithms and Technologies for Multispectral, Hyperspectral, and Ultraspectral Imagery XX. 90880H.
- A.H. Callaghan, G.B. Deane, M.D. Stokes, and B. Ward. 2012. Observed variation in the decay time of oceanic whitecap foam. Journal of Geophysical Research 117, 9 (September 2012).
- Darles, B. Crespin, D. Ghazanfarpour, and J.C. Gonzato. 2011. A Survey of Ocean Simulation and Rendering Techniques in Computer Graphics. Computer Graphics Forum 30, 1 (2011), 43–60.
- Goodfellow, J. Pouget-Abadie, M. Mirza, B. Xu, D. Warde-Farley, S. Ozair, A. Courville, and Y. Bengio. 2014. Generative Adversarial Nets. In Advances in Neural Processing Systems 27 (NIPS 2014).
- Isola, J-Y. Zhu, and A.A. Efros. 2017. Image-to-Image Translation with Conditional Adversarial Networks. In 2017 Conference on Computer Vision and Pattern Recognition (CVPR 2017).
- Koepke. 1984. Effective reflectance of oceanic whitecaps. Applied Optics 23, 11 (1984), 1816–1824.
- LeCun, Y. Bengio, and G. Hinton. 2015. Deep Learning. Nature 521 (May 2015), 436–444.
- Alireza Makhzani, Jonathon Shlens, Navdeep Jaitly, Ian Goodfellow, and Brendan Frey. 2015. Adversarial Autoencoders. (Nov 2015). arXiv:cs.LG/1511.05644
- Morel, D. Antoine, and B. Gentili. 2002. Bidirectional reflectance of oceanic waters: accounting for Raman emission and varying particle scattering phase function. Applied Optics 41, 30 (2002), 6289–6306.
- W.J. Pierson, Jr. and L. Moskowitz. 1964. A proposed spectral form for fully developed wind seas based on the similarity theory of S. A. Kitaigorodski. Journal of Geophysical Research 69, 24 (1964), 5181
Keyword(s):
Additional Images:
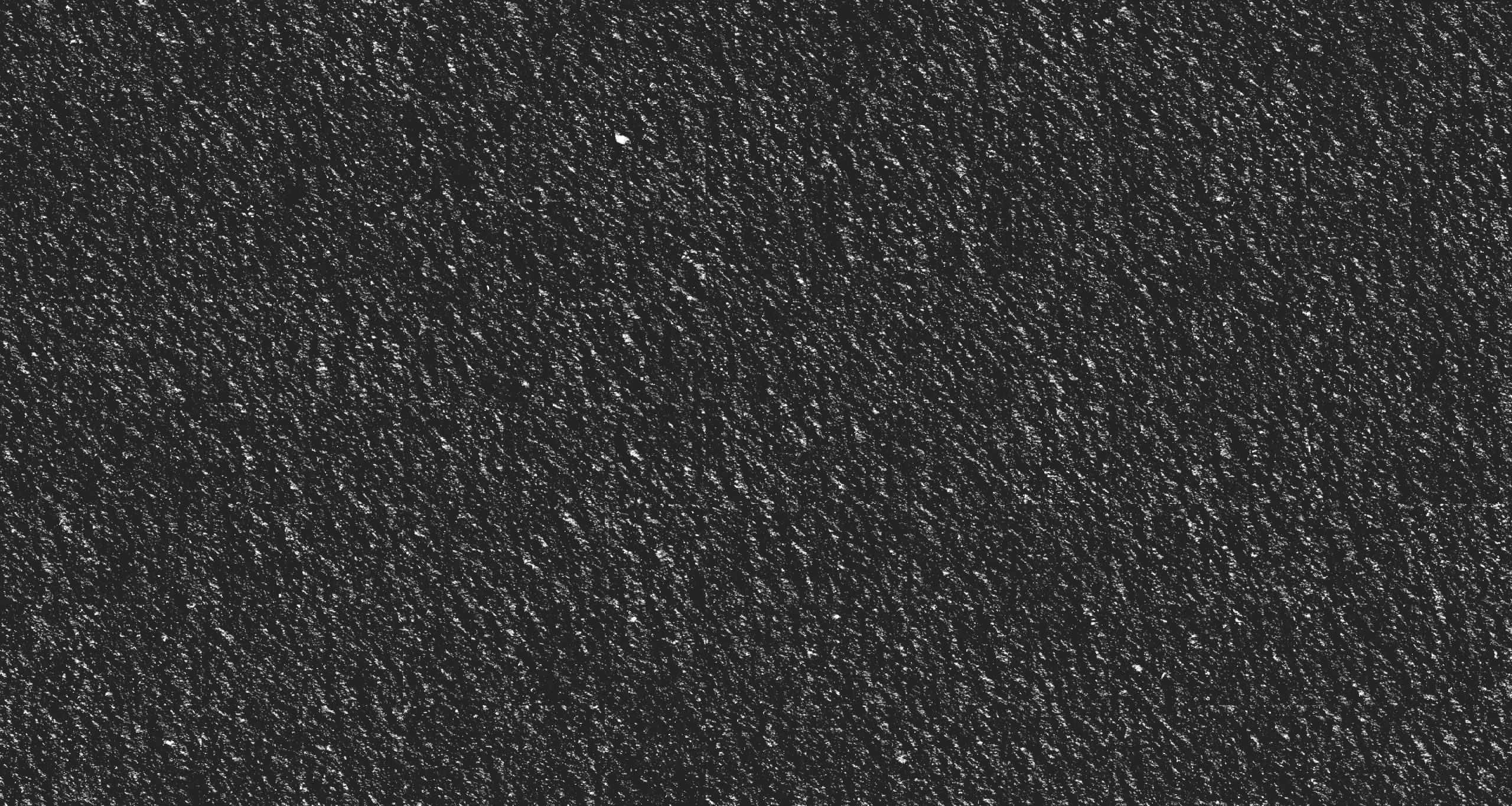