“Deep Learned Super Resolution for Feature Film Production” by Vavilala and Meyer
Conference:
Type(s):
Entry Number: 41
Title:
- Deep Learned Super Resolution for Feature Film Production
Presenter(s)/Author(s):
Abstract:
Upscaling techniques are commonly used to create high resolution images, which are cost-prohibitive or even impossible to produce otherwise. In recent years, deep learning methods have improved the detail and sharpness of upscaled images over traditional algorithms. Here we discuss the motivation and challenges of bringing deep learned super resolution to production at Pixar, where upscaling is useful for reducing render farm costs and delivering high resolution content.
References:
Steve Bako, Mark Meyer, Tony DeRose, and Pradeep Sen. 2019. Offline Deep Importance Sampling for Monte Carlo Path Tracing. In Computer Graphics Forum, Vol. 38. Wiley Online Library, 527–542.
Steve Bako, Thijs Vogels, Brian McWilliams, Mark Meyer, Jan Novák, Alex Harvill, Pradeep Sen, Tony Derose, and Fabrice Rousselle. 2017. Kernel-predicting convolutional networks for denoising Monte Carlo renderings. ACM Trans. Graph. 36, 4 (2017), 97–1.
Yifan Wang, Federico Perazzi, Brian McWilliams, Alexander Sorkine-Hornung, Olga Sorkine-Hornung, and Christopher Schroers. 2018. A fully progressive approach to single-image super-resolution. In Proceedings of the IEEE Conference on Computer Vision and Pattern Recognition Workshops. 864–873.
Additional Images:
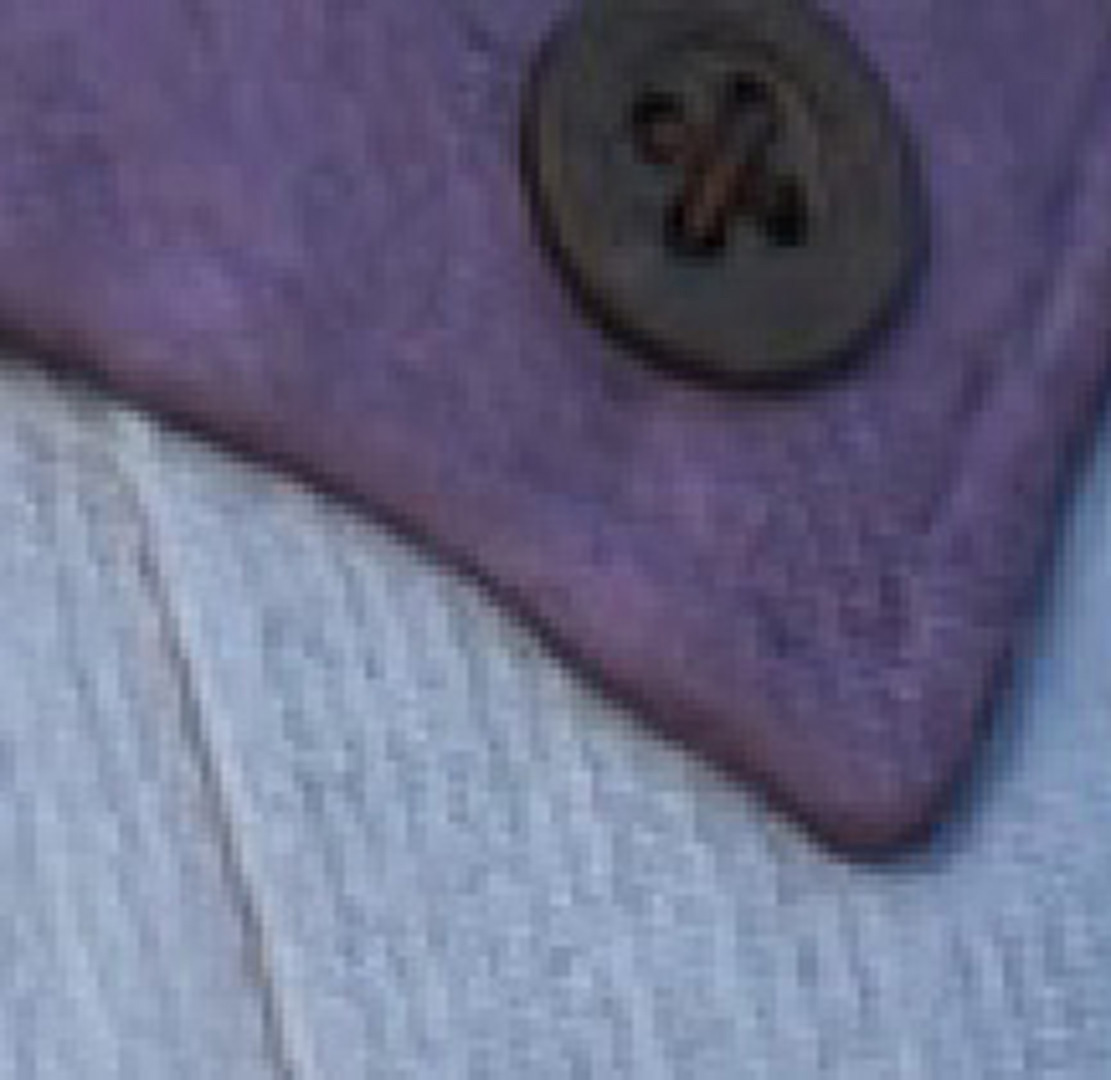

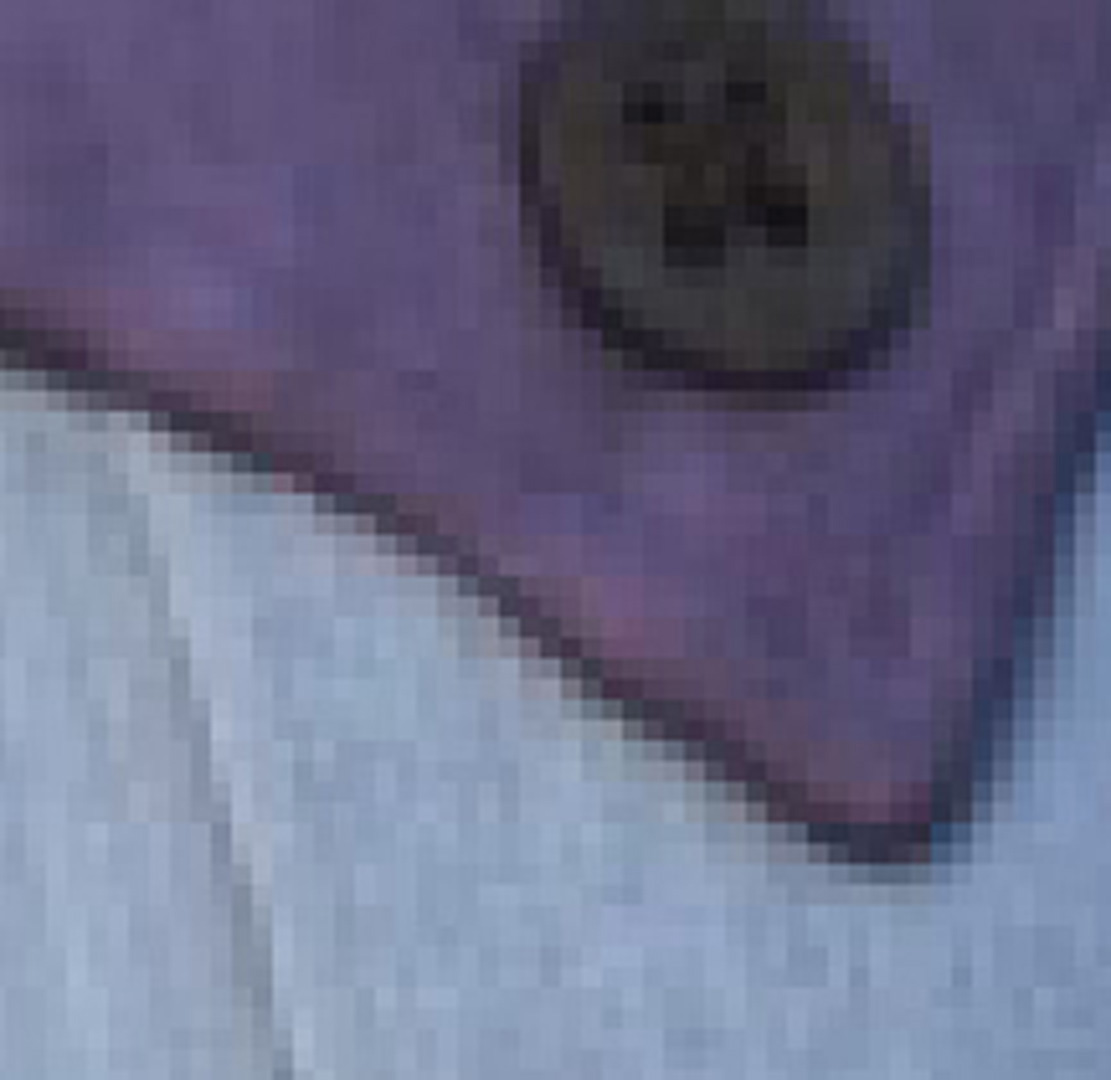

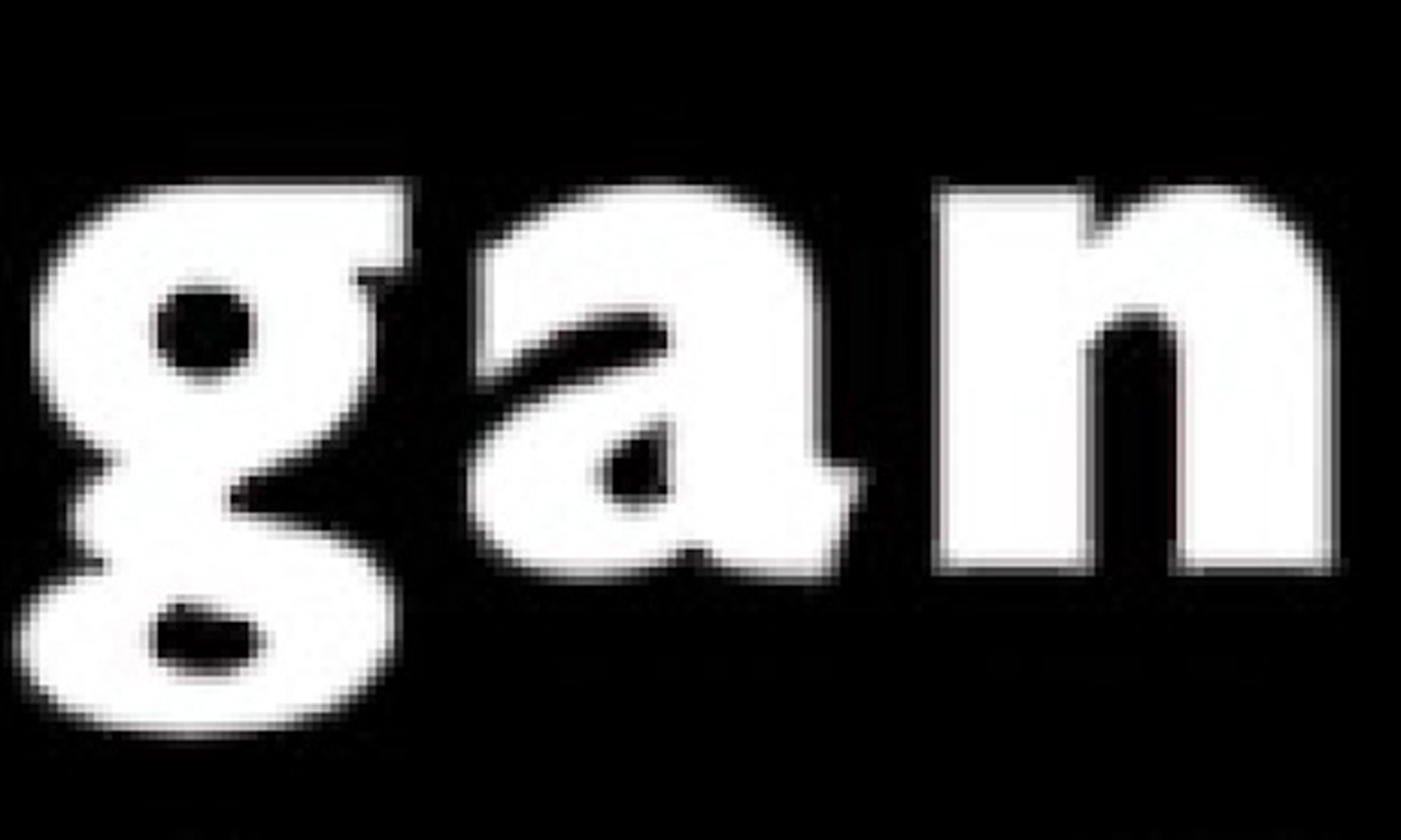
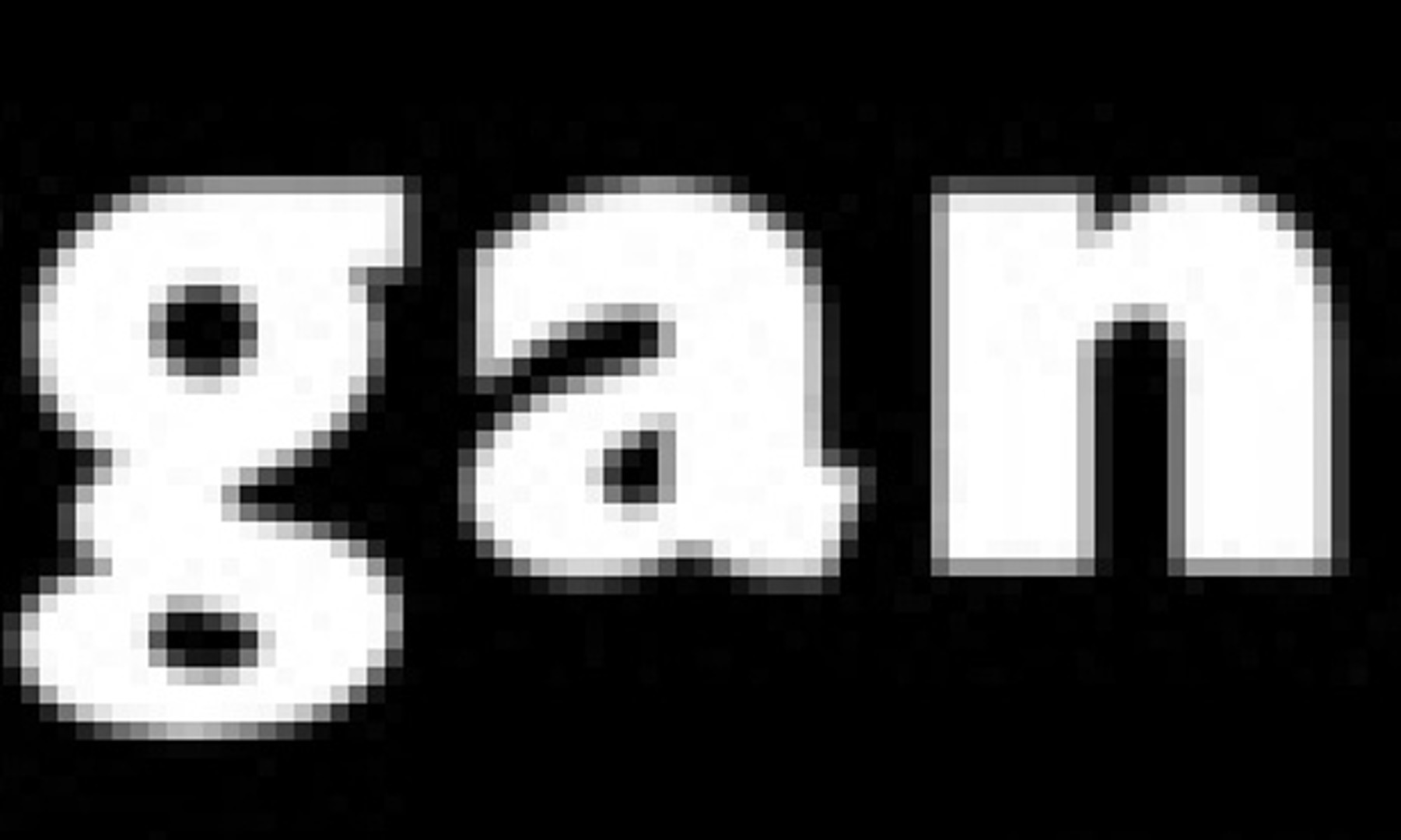


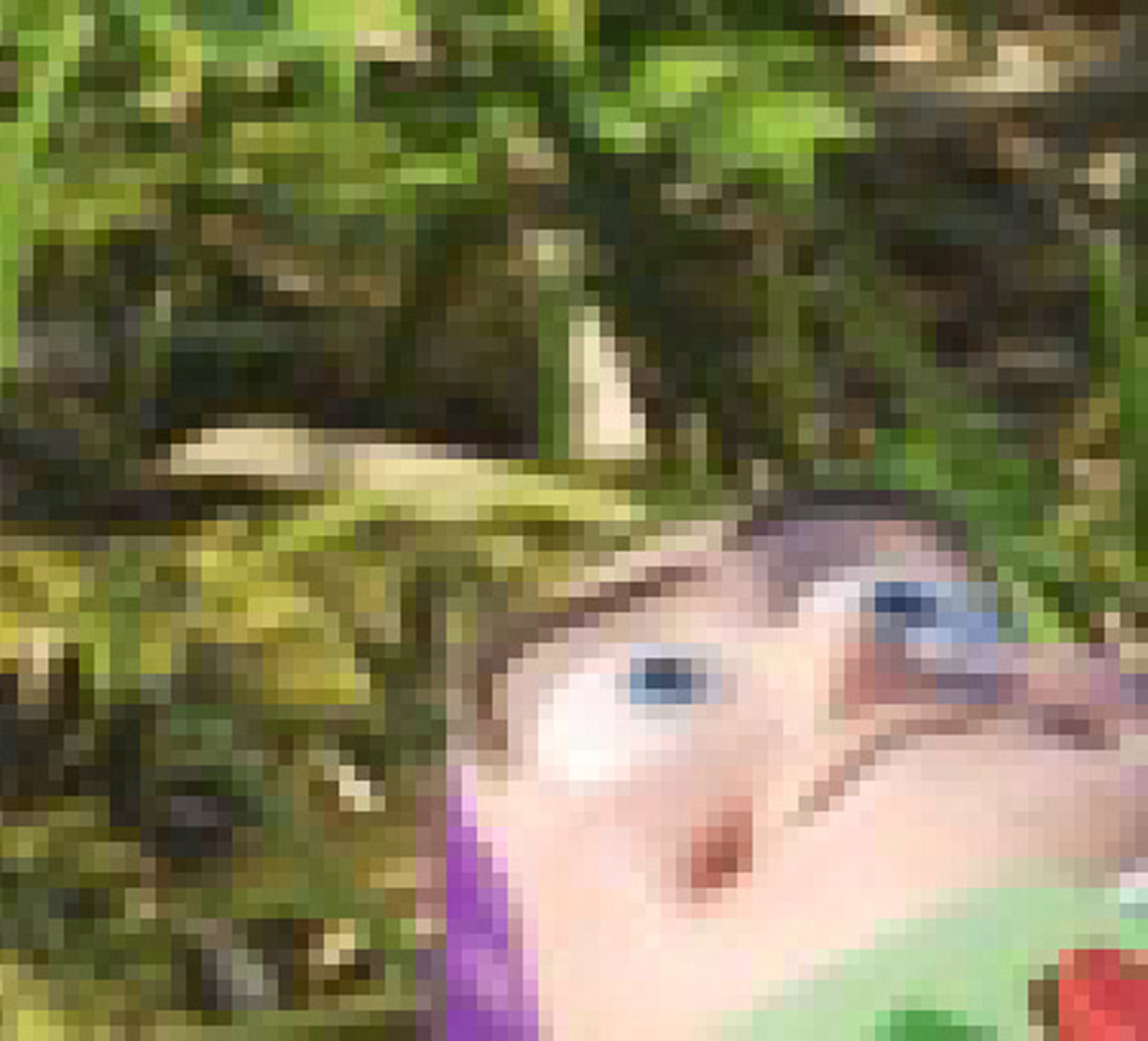
Acknowledgements:
The authors thank Christopher Schroers, Jeremy Newlin, Jack Zhao, Freeson Wang, Dominic Glynn, Eric Pearson, Mark Dinicola, John Halstead, and Steve May for contributing to this project.