“Belief propagation optical flow for high-resolution image morphing” by Lipski, Linz and Magnor
Conference:
Type(s):
Entry Number: 67
Title:
- Belief propagation optical flow for high-resolution image morphing
Presenter(s)/Author(s):
Abstract:
Over the last decade, considerable progress has been made on the so-called early vision problems. We present an optical flow algorithm for image morphing that incorporates recent advances in feature matching, energy minimization, stereo vision and image segmentation. At the core of our flow estimation we use Efficient Belief Propagation for energy minimization. While state-of-the-art algorithms only work on thumbnail-sized images, our novel feature downsampling scheme in combination with a simple, yet efficient data term compression can cope with high-resolution data. The incorporation of SIFT features into data term computation further resolves matching ambiguities, making long-range flows possible. We detect occluded areas by evaluating the symmetry of the flow fields, we further apply Geodesic matting to automatically inpaint these regions.
References:
Bai, X., and Sapiro, G. 2009. Geodesic matting: A framework for fast interactive image and video segmentation and matting. IJCV 82, 2, 113–132. Google ScholarDigital Library
Felzenszwalb, P., and Huttenlocher, D. 2006. Efficient belief propagation for early vision. In IJCV, vol. 70, 41–54. Google ScholarDigital Library
Liu, C., Yuen, J., Torralba, A., Sivic, J., and Freeman, W. T. 2008. Sift flow: Dense correspondence across different scenes. In ECCV ’08: Proceedings of the 10th European Conference on Computer Vision, 28–42. Google ScholarDigital Library
Smith, B., Zhang, L., and Jin, H. 2009. Stereo matching with nonparametric smoothness priors in feature space. Computer Vision and Pattern Recognition, IEEE Computer Society Conference on 0, 485–492.Google Scholar
Additional Images:
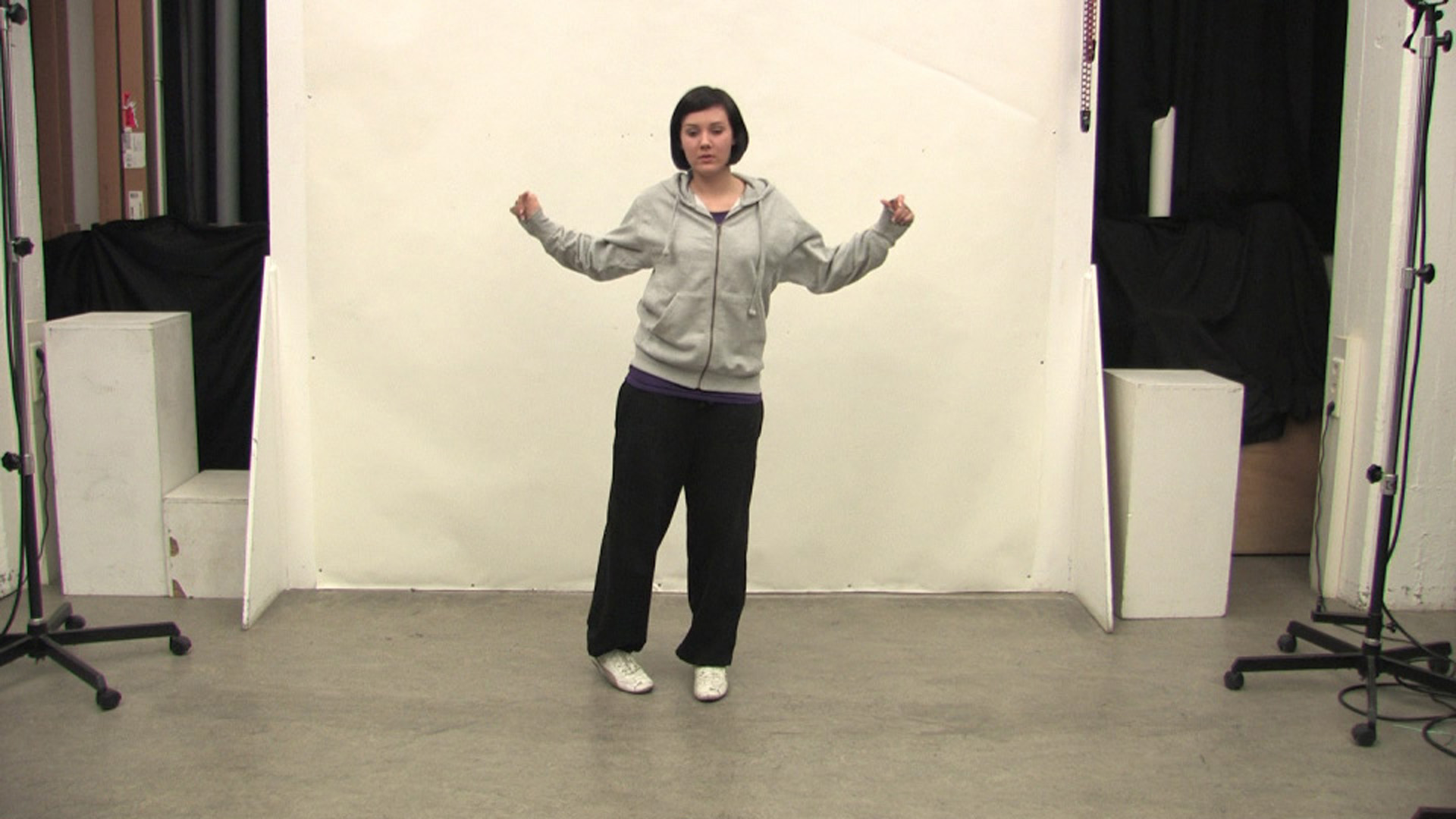
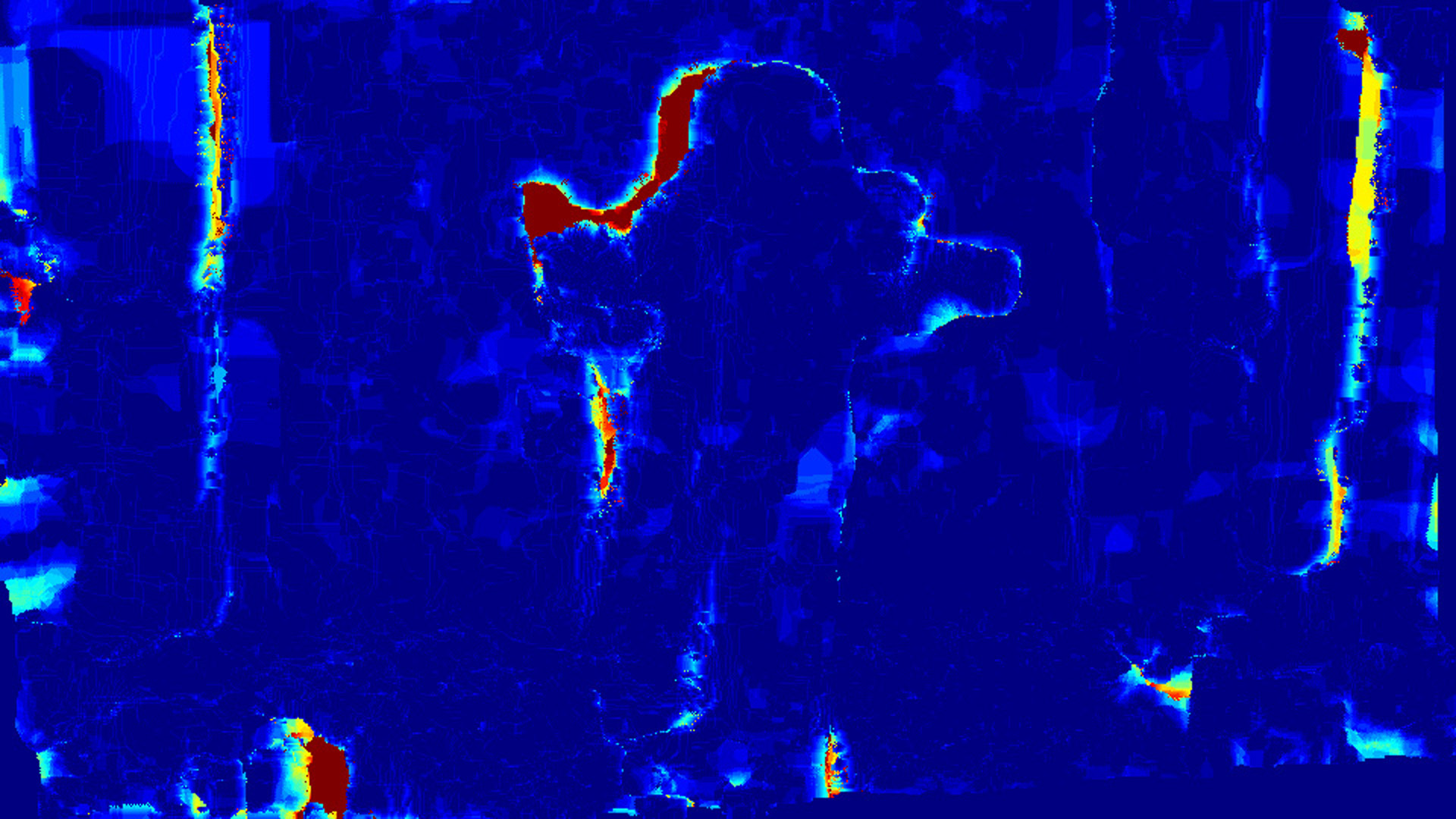