“Using Value Images to Adjust Intensity in 3D Renderings and Photographs” by Bailey and Grimm
Conference:
Type(s):
Entry Number: 057
Title:
- Using Value Images to Adjust Intensity in 3D Renderings and Photographs
Presenter(s)/Author(s):
Abstract:
We present a technique for manipulating the intensity values in a 3D computer generated image or photograph. Artists often make value sketches of a scene before beginning the final painting. This value sketch is based on the lighting of the scene, but is not simply a faithful copy of it. The artist uses the value sketch to experiment with the placement and tone values of large masses of light and dark areas. This results in increased coherency and focus in the final image, and also guides the viewer to the important elements of the image. The artist can do this because our eyes are sensitive to local luminance variation [Livingstone 2002]. Photographers and computer animators, on the other hand, do not have the luxury of simply changing the tone values of a rendering to create the image they want. Instead, they manipulate the lighting and the surface qualities of the scene objects to achieve the desired effect. A typical rendered scene in a high-quality animation film will have anywhere from ten to a hundred lights, placed in strategic locations. Similarly, photographers and film studios use spotlights and other special-purpose lighting to create better photographs.
We present an alternative method for adjusting a 3D computer generated image or photograph to achieve the desired tonal values. The user supplies a value sketch (which can be very rough) of the scene. Our system finds a mapping from the current shade values to new shade values that preserves the relative lighting and keeps as much contrast as possible. This mapping can be applied to the entire scene in order to attain the desired global tone values or to individual regions to attain the desired local variation.
References:
1. Comaniciu, D., and Meer, P., 1997. Robust analysis of feature spaces: Color image segmentation.
2. Livingstone, M. 2002. Vision and Art: The Biology of Seeing. Harry N. Abrams, Inc.
Additional Images:
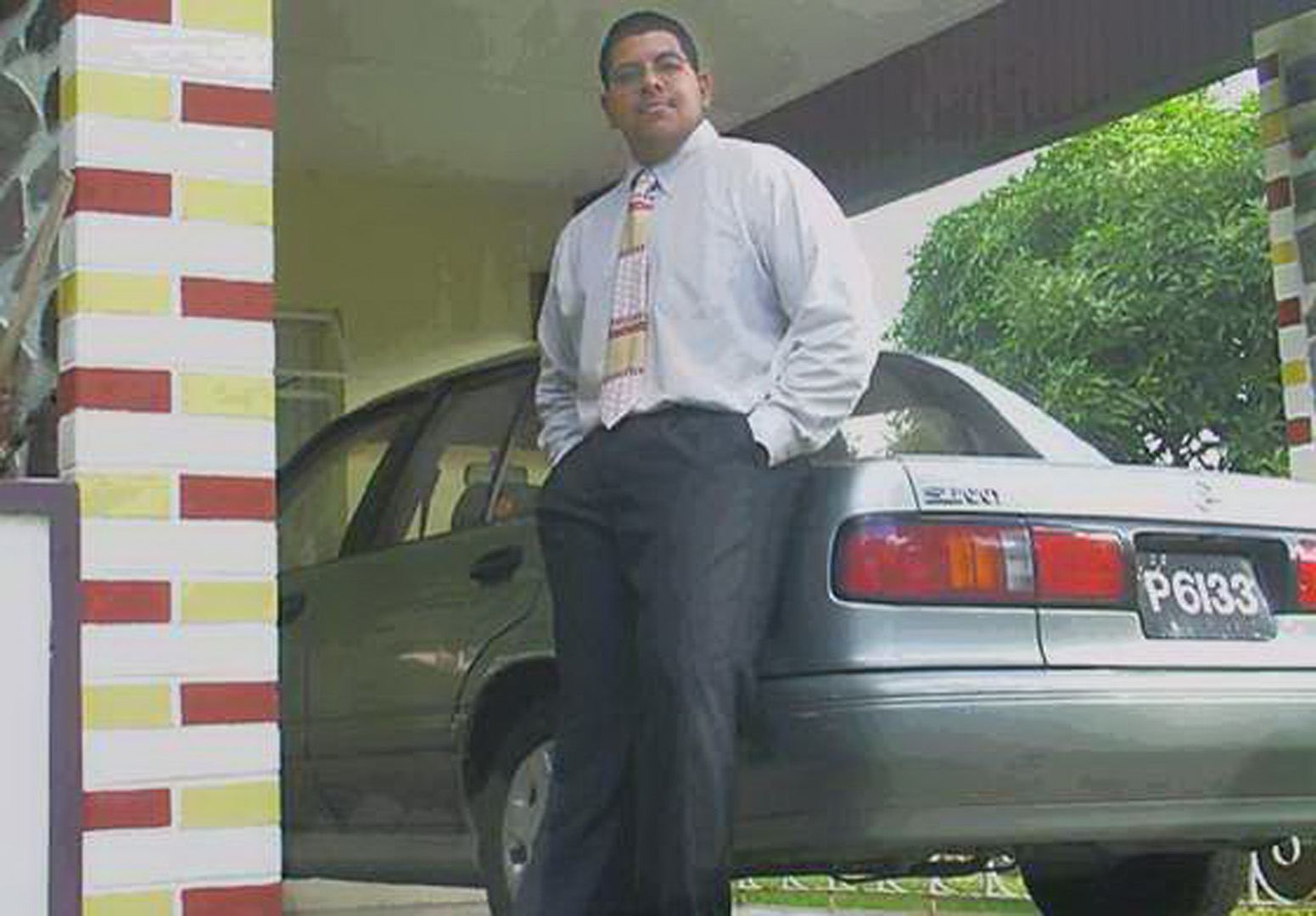