“Noise Reduction with Image Inpainting: An Application in Clinical Data Diagnosis” by Ke, Deng and Lu
Conference:
Type(s):
Entry Number: 88
Title:
- Noise Reduction with Image Inpainting: An Application in Clinical Data Diagnosis
Presenter(s)/Author(s):
Abstract:
For cytology, pathology or histology image analysis, whether performed by computer-aided algorithms or human experts, a general issue is to exclude the disturbance caused by noisy objects, especially when appeared with high similarities in shape, color and texture with target cell or tissues. In this paper, we introduce a novel model to reduce such type of noisy objects with large quantity and distribution in the microscope images based on deep learning and hand-craft features. The model experimentally reduces the false positives without effect on objects of interest for cancer detection. Moreover, it also provides much distinct images for human experts for the final diagnosis.
References:
Connelly Barnes, Eli Shechtman, Adam Finkelstein, and Dan B Goldman. 2009. Patch-Match: A Randomized Correspondence Algorithm for Structural Image Editing.
ACM Transactions on Graphics (Proc. SIGGRAPH) 28, 3 (Aug. 2009).
Geert J. S. Litjens, Thijs Kooi, Babak Ehteshami Bejnordi, Arnaud Arindra Adiyoso
Setio, Francesco Ciompi, Mohsen Ghafoorian, Jeroen A. W. M. van der Laak, Bram
van Ginneken, and Clara I. Sánchez. 2017. A Survey on Deep Learning in Medical
Image Analysis. CoRR abs/1702.05747 (2017). arXiv:1702.05747 http://arxiv.org/
abs/1702.05747
Keyword(s):
Additional Images:
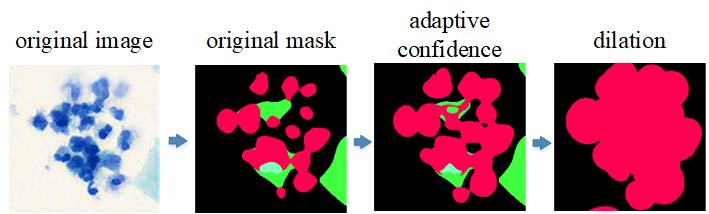
